Data Quality is important as the; technical, operational, commercial, legal, effectively all teams within your business will testify. The advancement of data and it’s integration into decision-making processes as small as what soap to order in your bathrooms to the enormous, should we purchase/insure/charter these ships?
This article can be summarised as stating the obvious: If you want to make good data driven decisions, you need high quality data, but much as I’d drive for a concise overview, there are some important variables to consider when applying this to your business, and here I’ll dive into these.
In this article I’ll focus on three of the most common metrics for assessing data quality: Coverage, Frequency, Accuracy. I’ll run through the pros/cons of these metrics as well as some of the secondary variables to consider within each,
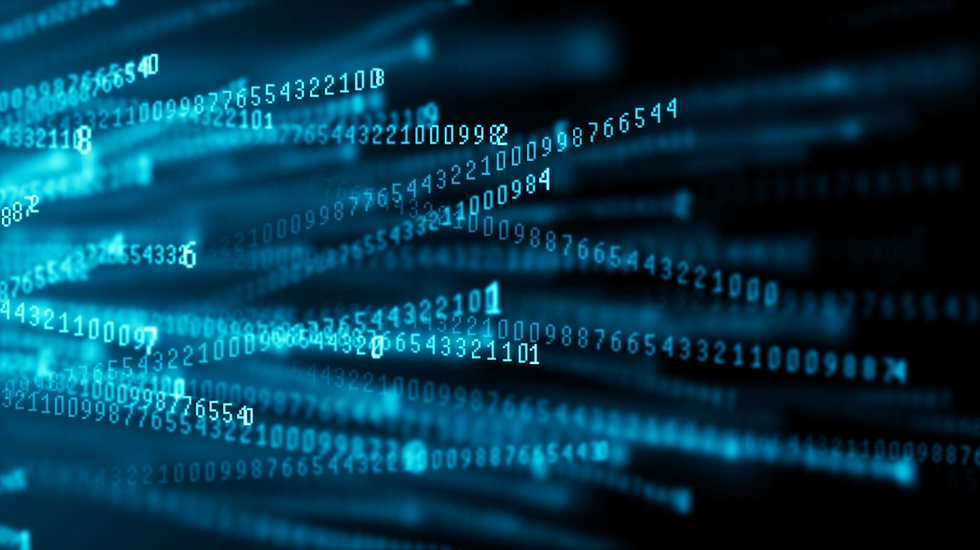
Coverage:
This is the simplest of the metrics, and looks at: What is the total universe according to this dataset?
To furnish this with a couple of examples from the last few months:
· Coverage of AIS is the total number of unique MMSI numbers within the dataset over a specific period
· Coverage of vessel characteristics looks at two variables: the number of vessels within the dataset and the number of fields available, however this can be more narrow if you have a specific target, for example the number of container vessels and the coverage of container specific fields (eg TEU)
· Fill rates of a vessel database – how many of the fields are populated for the relevant vessesl.
· Coverage of a Geospatial port database is the total number of ports, terminals and berths
To start with the advantages.
Coverage is a simple metric which you can use to easily filter out datasets which are not relevant. If you target the RORO market, and this dataset has a total number of RORO ships which is significantly below what you understand it to be, that’s a strong early indicator that one of you is incorrect. Which could either be a huge boon to your business moving forwards, or a warning that this dataset is not going to add much value to your business.
Additionally, it’s easy to work out. Aggregate figures are simple to calculate, if not provided up front by the supplier as part of the sampling/trial process.
To look at some of the drawbacks.
Coverage relies on a comparison point to make a solid judgement, if you’re not sure what the total size of the dataset should be, it’s difficult to make any form of reasoned assessment.
Coverage as a standalone judgement also makes no quality assessment. A supplier could have a fleet of 400000 vessels, a port database totalling 25000 ports and a vessel database with 1500 fields, but to provide an extreme example, if 10% the data is real and the rest is poorly derived outputs, the dataset will very likely cause more problems than it solves.
Frequency
Frequency looks at the rate at which the data is updated, for example:
· The available delivery of real-time AIS
· The update cycles for new vessel data
· How many times annually port databases are updated
· CII calculations
· How frequently vessel ownership details are changed
Here the benefits and the drawbacks are related, as it will massively depend on the dataset that you’re looking for.
If you’re an organisation that runs a selection of real-time trading applications with AIS data as an input, a supplier which can provide these 12 hourly or daily simply isn’t going to address your need.
In contrast to this, many organisations will use a vessel database with a quarterly update cycle, as the dataset is static enough that a daily feed is simply a waste of time and processing power.
As a result of this, the frequency metric is very context dependent, and one that your teams will apply as necessary.
Accuracy
In no small part, the most difficult metric to calculate, but fundamental to the evaluation of any dataset.
Examples of this include:
AIS Data:
Accuracy is are the vessels where the data says they are at any given time. This metric should consider:
· Existing knowledge of specific vessels (spot testing)
· Aggregate testing of vessels obviously out of place (on land/travelling thousands of miles in minutes etc)
· Testing of the number of duplicate positions, and subtracting this from the number of positions.
Vessel Data
· Comparing known fields against the data (is this the right engine designation for a vessel we recently installed an engine on, for example)
· Checking the aggregate numbers against known data from trusted sources (brokers, customers etc)
Ownership Data:
· Comparing the ownership data provided against known examples
· Spot checking company details against public registries
· Comparing against free sources (www.equasis.org)
The benefits of such checks should be apparent, they provide tangible live examples of data quality and can be used to extrapolate overall feedback for of the datasets. This type of quality assessment will be fundamental to most purchasers of data, and will be used to guide decision making.
The first challenge of this methodology arises when looking at the comparisons. They require an accurate source. If this accurate source is not available quickly or easily it can massively slow down the process.
And on the topic of slowing down, this process, due to its complexity and individuality for each dataset is time consuming. This often occupies developer and analyst time, on top of their existing commitments.
Conclusion
As covered above, these three metrics are widely used by organisations in conjunction with more specific assessments for their individual needs. As you can see from the steps, the level of time/resource/expertise required for each step increases the further into the process you go, and if you’re assessing multiple datasets at any one given time can become a huge project.
Some buyers will use the above processes as stage-gates, looking first at coverage to determine whether it’s worthwhile progressing to the more complex analysis, but others will choose to run the full, thorough, evaluation on all datasets, as what they may lack in the coverage they make up for in accuracy or frequency.
Ultimately, the decision is yours and your teams, as to which works best, and will likely be coloured by the available time and resource, alongside the value of the dataset to your overall objectives.
Final notes
At Maritimedata.ai we seek to connect you with suppliers of data, analytics, and research services relevant to your requirement, and add a step to the start of this process by taking your RFI, understanding the exact objectives you have for these tools, and filtering out suppliers which can/can’t support you. If you’re interested in understanding the process please reach out to us here.
Comments